Barriers to ‘Personalized Medicine’
Since the first human genome was sequenced in 2003, the amount of next-generation sequencing (NGS) data has increased exponentially while costs have continued to decrease (Figure 1). Along with increasing accessibility to NGS data, there has also been a steady increase in genomic knowledge-base, algorithm development, and computational resources.There have been several successful cases of ‘personalized medicine’ demonstrating its potential; however, the promise for ‘personalized medicine’ has not yet been fully realized (Figure 2). A few major barriers to progress have been: i) the availability and reprocessing of open access data, ii) algorithm standardization, including benchmarking, and iii) a lack of a computational infrastructure that is built to enable a broader cross-section of researchers to access integrated data and execute bioinformatics workflows.
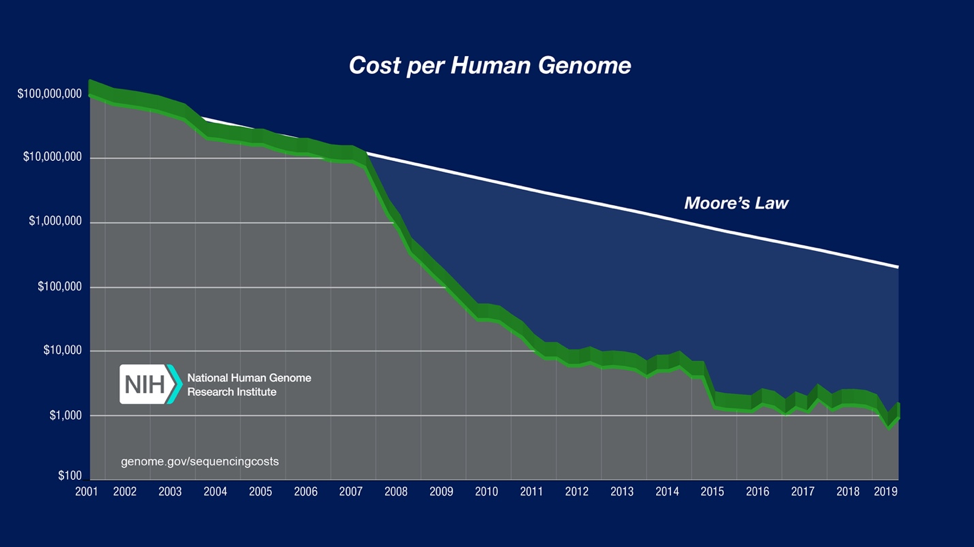
These barriers are not without challenges. While scientists may relatively easily obtain the necessary credentials to acquire Open Access data, there are additional processes required to fully utilize all available datasets. For example, if bioinformaticians desire to integrate samples across various sources, they need to ensure that the samples were processed using the same workflows in order to remove background sources of variation that may be introduced by the use of different methods. This process requires shared data access, cleaned and curated metadata, and standardized workflows. While the scientific community has settled on some standards, there are countless numbers of algorithms available for each step in bioinformatic workflows. For example, there are now more than 30 variant callers and 40 functional annotation tools creating ‘where to start?’ and ‘what are best practices?’ dilemmas.
Adding to the complexity, the optimization challenge continues to shift based on who is optimizing the problem. Some accepted performance metrics include precision/recall, sensitivity/specificity, run time, and compute resources. Furthermore, the selected metric often changes the optimal algorithm. While experienced informatics specialists have the skills to navigate many of these challenges, the much larger community of life sciences researchers are excluded from executing modern bioinformatics workflows on their own. If personalized medicine is going to become a reality, the life sciences industry must solve how the broader research community can participate.

New Approaches Needed
Currently, if a researcher wants to utilize NGS data, there are two primary options: either the computational infrastructure and analytic solutions are developed internally or the analysis is outsourced to a third party vendor. On one hand, developing infrastructure and analytics internally is expensive, time consuming, requires specialized talent, and is not guaranteed to succeed. Internal solutions may also require ongoing software developer and engineering support to ensure solutions remain performant, secure and updated. On the other hand, if researchers outsource their analytic solutions, they lose control of the analysis methods and the timelines required to complete the job. Outsourced solutions are completely dependent on the outsourcing company’s bandwidth and often turnaround times exceed multiple weeks. Additionally, a premium is placed on outsourced analytic services driving up price because there is limited competition in the space. Neither of today’s solutions democratizes access to the data and analytics in a way that can be leveraged industry-wide.
A new solution is needed and should take the form of a platform that spontaneously provisions and configures the necessary infrastructure. Ideally it would serve up solutions to users in a UI designed to be used by both informatics specialists and working biologists. Further, this on-demand infrastructure should be task optimized, reproducibly designed, and user-controlled. It’s a new model to put the power and control in the hands of the masses so ‘personalized medicine’ can be realized and not depend on a handful of specialists that are already overwhelmed.
A Computational Framework for Analytic Standardization
A computational infrastructure built for broad life sciences data analysis must reliably handle the comparison between varied approaches and enable the development of standards. This is particularly important given that rapid pace of technology development. Without extensible infrastructure that can handle diverse workflows, identify best solutions, and standardize each step for larger teams, researchers are just laying down ground fire over and over, never locking onto an intended target.
The Catalytic Enterprise Platform was built to address these exact problems. Research data is easily connected through data lakes or popular ECM solutions so that exposure to analytics services happens with a few simple clicks. Comprehensive bioinformatic workflows from raw data ingestion to report generation, including figures and tables, are developed and published with an easy to navigate user-interface designed to be executed by specialists and generalists alike. The Catalytic scientific team continuously measures and benchmarks novel analytic solutions to help researchers make more informed decisions about data analysis choices. Data analysis pipelines on the Catalytic Platform are designed for easy customization and will accommodate specialized cases. Parameter settings and filter options for each workflow can be exposed to the specialist enabling granular tuning of the pipeline. Once pipeline settings are established by a specialist or by Catalytic’s Data Science team, the customized workflows can be locked and shared with team members and collaborators. Importantly, all bioinformatic solutions on the Catalytic Platform are optimized for efficiency, parallelized for scale and secured for privacy. The result is that not only can the masses execute NGS data analysis projects, but they can do so confidently and at a scale that will accelerate our progress toward a personalized medicine future.
As the industry capitalizes on Big Data for health therapeutic and life science applications, Catalytic is here to provide standardized and validated analytic solutions. Our team of computational biologists, data scientists and software development engineers have built a new type of computational infrastructure that is controlled by the very researchers that are tasked with data analysis workflows. Our team is available to help compare available bioinformatic solutions, discuss best practices and assist every step of the way until all researchers are running customized workflows on the Catalytic Platform. Our mission is to remove the analytic bottlenecks that currently restrict democratized use of NGS technology.