“It was the best of times, it was the worst of times, it was the age of wisdom, it was the age of foolishness, it was the epoch of belief, it was the epoch of incredulity, it was the season of light, it was the season of darkness, it was the spring of hope, it was the winter of despair, we had everything before us, we had nothing before us…” (Dickens, A Tale of Two Cities, 1859)
Best of times or worst of times?
Dickens could have been writing about the state of the life sciences industry in 2022. There have never been more companies, more capital, and more exciting research and development programs than right now. Over the past decade, the number of new companies formed each year in the life sciences sector has doubled from approximately 200 to 400 per year. Moreover, the average amount of money raised by those companies over the past five years has increased from $68.6 million to an eye popping $198.1 million. It has been the best of times and the spring of hope for companies, investors, and, most importantly, patients.
However, macroeconomic and geopolitical turmoil has created rapidly declining corporate valuations and a radically transformed industry dynamic. An unprecedented number of public and private companies are making pipeline differentiation increasingly challenging, the competition for research and data science talent fiercer, and the prospect for future capital raises precarious. Unfortunately, these risks are likely to persist for several years and be managed in an environment of flatlining translational success. While 2021 was a banner year for approval of new molecular entities, with 50 gaining a green light from the FDA, that number alone doesn’t tell the entire story. As figure one demonstrates, new drug approvals per $1B of R&D spent has not improved for decades. Thus, while financial metrics surged during the spring of hope, R&D efficiency remained in a long winter of despair.
Figure 1.
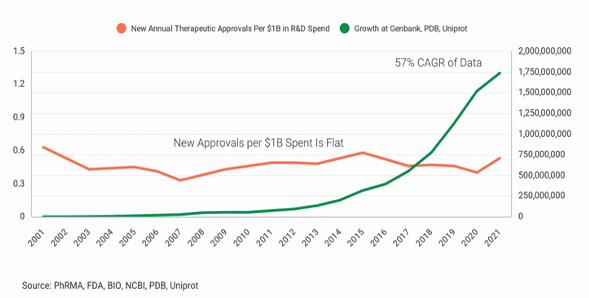
What does any of this have to do with research informatics?
Industry dynamics have changed, so the way companies think about their organizational structure, cost control, and R&D efficiency must also change. Senior leaders can no longer develop strategic plans looking in the rearview mirror, assuming the favorable sector dynamics of the past decade will persist. Capital will be tighter, competition for partnerships more intense, and the need to quickly achieve research milestones greater. Assuming layoffs and pipeline cutting aren’t the desired plan, a rethink of how to operate is imperative because companies will have to produce more research faster and with less capital. Implementing new, or upgrading old, research informatics systems is an investment companies can make today in preparation for a less indulgent future. It may sound delusional that research informatics will increase the probability of organizational success, and it’s certainly not a stand-alone panacea, but here’s why it’s true.

Over the past decade, research methodologies that produce experimental data have profoundly changed. Most laboratory instruments have digitized (those that haven’t are well on their way) and now produce large complex data sets faster than ever. Likewise, the downstream analysis of digital data has moved in silico, creating the opportunity to build scalable pipelines to keep pace with increasing data loads. This means that more of the core workflows that yield unique scientific insights (corporate assets that can be monetized) are computational and will rely heavily on purpose-built digital infrastructure to scale. In other words, as data production and analysis digitize, so must a company’s research infrastructure. Thus far, however, the industry has been slow to realize the mismatch between the type of data it produces and the informatics infrastructure tasked with making sense of it.
We, of all industries, should know better. One of the basic principles of biology dictates that a molecule’s structure will determine its function. For example, mRNA is a form of information that encodes instructions for making a protein. A ribosome is tasked with deciphering that information and turning it into a protein. Thus, in this example, mRNA is “data,” and the ribosome is “infrastructure” exquisitely designed to process this specific type of data and produce an output. If a receptor tyrosine kinase was tasked with translating mRNA it would fail, and the information encoded in the transcript would be lost. Structure determines function. Yet, the structure of research informatics today is not exquisitely designed to process digital research data and does about as good a job as a receptor tyrosine kinase would if attempting to translate mRNA.
So what? A data/infrastructure mismatch results in slow and capital inefficient research processes that don’t deliver enough high probability clinical candidates. In the best of times, this mismatch is problematic, leading to low R&D ROI, and in the worst of times, this mismatch could be the difference between success and insolvency. The life sciences industry can do a lot better.
Research informatics platforms will become key competitive differentiators.
As the future of discovery research marches relentlessly toward producing digital assets, in silico analyses, machine learning driven predictions, and expert systems, senior leadership must become more fluent in the technology lexicon. The pace of research progress is always throttled by how fast your team can ask and answer scientific questions. That means your research programs will only move as fast as your systems can deliver answers to your team. If your researchers can’t instantly access information, analyze, query, and interpret the results without struggling with technology and tools, you are wasting time, burning capital, and falling behind the competition.

It’s senior leadership’s responsibility to create an informatics strategy and drive transformation from the top. Your team’s input can influence the “shape” of your platform, but if you rely on your team to develop a strategy or inform executives about what is needed, failure is inevitable. If left to team members, senior leadership will most often hear “we don’t need anything else right now” or receive a request for a command line tool running in AWS that can only be used by a few researchers or be pitched a loosely defined project to build something internally that doesn’t address core research bottlenecks. This is to be expected because it’s the environment team members work in and their job is not to develop forward-looking strategic roadmaps that lead to a more competitive company.
Digital fluency is not hard to learn, but without it, how will leadership develop strategy, know what questions to ask, hold teams accountable or conceptualize a roadmap to move ten times faster? Understanding tech stacks, or in this case, bioTech stacks, is an excellent place to start because it forms the foundation of a sound research informatics plan. Tech stacks are simply a collection of technologies that work together to deliver the desired business outcome. The importance of a well-designed tech stack cannot be overstated because it enables all departmental workflows to connect, accelerate, scale, adapt, and remain secure. For clarification, procuring EC2 instances in AWS managed by in-house data scientists to run bioinformatics pipelines from the command line is not a corporate research department tech stack but rather a siloed workflow that might be part of a stack. A tech stack for corporate research departments should enable ALL workflows from pre-discovery through preclinical development (whether literature or data) where ALL team members can work in a shared computing environment.

Today, computational workflows are capped by the limited availability of data science talent that is expensive, hard to find, and mostly wasted executing analyses that biologists can perform given the right user interface. Companies that implement highly performant tech stacks in their research departments will produce significant efficiencies in how much data is processed per unit of time, how many team members can perform analyses, and how quickly future capabilities can be added. Teams will be able to collaborate from anywhere, reason over larger information spaces to increase decision accuracy, better manage research budgets, and, most importantly, achieve research milestones faster. In other words, those companies that implement performant tech stacks will be more competitive across every part of the research cycle regardless of market dynamics. Smaller teams will do more with less and become tomorrow’s winners.
10 Must-Haves in a research informatics platform.
Life sciences companies are data-driven decision-making machines. At least they should be. In fact, many companies have some form of “data-driven decision-making” in culture and value statements. Performant informatics platforms are a powerful way to align aspirational goals with execution capability so companies can walk the talk when enabling data to drive decisions. In addition, delivering better informatics capabilities to a research team is among the most effective talent retention strategies a company can implement to keep the best and brightest in their roles. Nothing burns out a researcher faster than struggling with outdated technology that slows or blocks the ability to get work done.
Delivering specific and pre-defined research outcomes requires each company’s tech stack to be customized. However, for most research departments, a foundational requirements list will be standard across companies regardless of scientific focus and stage of pipeline development. Figure 2 illustrates how a well-designed informatics platform can capture workflows, grow a company’s knowledge graph, and provide a computing interface to enable new scientific insight creation. The goal is to create a fly-wheel effect that amplifies collective corporate intelligence and decreases the time required to meet milestones. The following ten must-haves will ensure that an informatics platform will be performant for current workflows and adaptable to the future emergence of new and better research methodologies.
Must Have Capabilities
- No code user interface that empowers the entire team to work in one shared environment
- Ingestion pipelines that upload and harmonize diverse content and data formats at scale
- Comprehensive coverage of all required data analytics and bioinformatics pipelines
- BioNLP driven scientific search and text mining
- Central knowledge graph that updates, learns, and predicts as research is conducted
- A software architecture that is rapidly composable and configurable to new capabilities
- Workflow creation that enables biologists to execute complex data analyses
- In-platform communication and collaboration at the point where work is happening
- Connectivity and integration with existing and future applications (ELNs, LIMs, SDMS)
- Single-tenant private cloud hosting

Figure 2.

Step one to implementing a research informatics platform – build or buy?
Once the decision to implement a new research informatics stack is made, the first step is to decide whether your company should build it internally or buy a solution from providers. This decision must recognize that getting it right requires multi-disciplinary software engineering expertise that most life sciences companies don’t have and are unlikely to attract as full-time employees. Great software engineers want to work for software development companies with a software engineering culture; thus talent acquisition is challenging for the “go it alone” path at a life sciences company. A significant misunderstanding in the life sciences industry is that bioinformatics, computational biology, and other data science roles can be hired to build software and systems. Building tech stacks is not what these roles do, and tasking them with the work will fail. In other words, data science talent should be computing on your informatics platform, not wasting time trying to build it. Finally, building a performant stack requires significant capital and a sustained effort over time that calls into question whether a life sciences company should ever use its cash to build software.
As mentioned previously, a tech stack is a group of technologies that work together to deliver a company’s desired outcome. Inherent in this definition is that many disparate and complex compute resources must be integrated to deliver the desired result of a performant informatics platform. We are fortunate to have extensive computing resources available today (thanks to Cloud providers), but we are seriously limited by computational complexity. In reality, despite availability, computing hasn’t gotten any easier for researchers that need to ask and answer biological questions that move workflows forward. The skill sets and capabilities required to build a performant research informatics platform, secure it, and maintain performance include:

Surveying the diversity of expertise needed to build scalable fault-tolerant computing platforms and the complexity of the required capabilities argues strongly for life sciences companies to join other industrial sectors that rely on outside partners that provide their technology stack(s).
When is the right time to implement a research informatics platform?
A popular proverb states, “The best time to plant a tree was 20 years ago. The next best time is today.” When implementing a new research informatics platform, this proverb means that if you want success and growth in the future, the best time to act is now. Companies should not try to boil the ocean and implement a comprehensive end-to-end informatics platform that can handle all research workflows on day one when getting started. This type of strategy will only become mired in complexity and empower the naysayers who will create opposition at every turn. Instead, a well-defined core need should be the starting point. A sound strategy is to define a specific research need and align that need to a particular new component of a tech stack that will solve it. Showing progress will create motivation and build momentum. This post isn’t focused on the how but rather the why, so we won’t go into further detail on this topic here. The main point is to get started, start small, gain momentum, and don’t let perfect be the enemy of great. A research informatics tech stack will never be completed because new capabilities will always emerge that should be integrated. Still, without a solid foundation in place, companies will be unable to take advantage of better research approaches when they come.
Measuring the value derived from performant research informatics platforms.
The topic of how to measure the return on investment (ROI) of software systems is a rabbit hole that goes deep. Conceptually, it’s simple. First, define what outcome you want your software system to deliver to your organization (Return) and then determine the total cost of implementing the system (TCO). ROI = Return ÷ TCO. Determining TCO is straightforward, but determining Return is complex. Returns could come from cost reductions, speed of executing workflows, the throughput of data analysis per unit of time, the number of therapeutic targets validated by the research department, etc. The determination of what Return a company is seeking from new research informatics capabilities is numerous and will depend on the specifics of each company. Regardless, determining the desired Return is a critical exercise because it’s what will be measured to ensure the informatics stack is delivering on its promise.

Perhaps a good place to start is reducing the research process to its most basic level. Most research in life sciences is performed to generate a unique insight about a target, properties of a molecular entity, genotype/phenotype relationship, mechanisms of action, drivers of pathophysiology etc. These unique insights hold the ability to create economic value for the company and deliver benefit to a patient. There are only several ways a life sciences company can monetize its work and fund its business. It can license intellectual property, form development partnerships around technology or therapeutic candidates, raise money from investors based on the promise of its technology or pipeline, or be acquired by a company that wants to own its technology or pipeline. All of these potential monetization paths are driven by the fact that the research department produced a unique insight about something valuable. Of course, other groups in the organization (clinical, regulatory, manufacturing) are required to mature the asset, but there aren’t assets to mature without productive research. Unique scientific insights are the fundamental unit of value companies are trying to produce at scale.
Given unique research insights are the assets that drive monetary value, it stands to reason that the Return on a research informatics platform could be quantified in terms of how many unique insights are produced over a set period given fixed team size and budget. Comparing this number to the historical baseline created with old processes will determine the Return a company receives from its investment in a new tech stack. Once this number is known, prior development success rates or partnership success rates can be applied to calculate the economic return expected by the higher insight generation rate. Of course, unique insights are not overnight epiphany events made by lone researchers but are generated by teams conducting multiple experiments and analyses over time.
Figure 3.

Let’s put some numbers to this concept using historical probabilities of success for R&D programs. Figure 3 shows the average probability of success for each stage of an R&D program from target discovery through FDA approval over the past twenty years. These probability of success numbers are for all therapeutic classes combined. Starting with the probability of success averages a calculation can be made to determine how many new therapeutic targets plus lead molecule combinations are required by a research department in order to produce one approved therapeutic. The calculations show that approximately 18 therapeutic target/lead molecule combinations are required to generate a high probability of one therapeutic approval at the end of a full R&D cycle. Thus, for starters, a research team and it’s systems need to generate a sufficient number of unique scientific insights to generate at least 18 therapeutic target/lead molecule combinations at the top of the funnel. But, if that same team has a better informatics platform that enables the generation, analysis and interpretation of far larger amounts of information and could double the number of new target/lead combinations while probability of success remained fixed, then the number of approved therapeutics would double along with the number of potential monetization events along the way.
However, and more importantly, what if a performant informatics system was implemented that enabled the same research team to improve their decision-making accuracy because they could reason over larger information spaces and raise the collective intelligence of the team leading to just a 10% increase in probability of success at each stage? Figure 4 shows that same team could double the number of therapeutic approvals based on the same number of 18 target/lead combinations! If that same team improved probability of success by 10% at each stage and doubled the number of new target/lead combinations it would nearly quadruple the number of new approvals.
Figure 4.

Applying financial value to the doubling of assets that can be monetized or the doubling of therapeutic approvals provides a solid rationale for implementing research informatics platforms that scale the number of unique insights a research team can generate. The attractiveness of this approach is that leadership doesn’t have to wait for product approvals in the distant future to know if the ROI of its investment in a new research informatics platform paid off. Tracking the number of unique insights generated and growth in the preclinical pipeline can be measured on much shorter timeframes providing near-term tracking and course correction if required.
Plant that tree today.
This post starts with a Dickens excerpt that aptly describes the state of the life sciences industry today and will end with the opening lyrics to Bruce Springsteen’s Cover Me.

The old world of easy money, inefficient R&D, and bloated organizational structures will only make it tougher to compete and thrive in the current industry dynamic. Companies that lead from the top, develop strategies to implement performant research informatics platforms, and put realistic roadmaps in place to get started will have distinct advantages over those that don’t. The future of life science R&D will continue to move toward methodologies that create digital data, automated computational workflows that uncover unique scientific insights, and powerful informatics platforms that entire departments compute on to raise the efficiency and collective intelligence of the company. Get started and plant that tree today. The informatics canopy that results will provide the cover required to remain competitive in any future industry environment.